Chapter 1. Introduction
Certainly, in today’s ever-evolving world of sports, where every pass, every goal, and every move is scrutinized and celebrated, the influence of machine learning algorithms is emerging as a game-changer. Moreover, as soccer enthusiasts and betting aficionados alike seek the holy grail of accurate predictions, the fusion of artificial intelligence (AI) and sports is paving the way for a new era of precision. Welcome to the exhilarating journey into the world of “Understanding Machine Learning Algorithms in Soccer Predictions.”
1.1. The Growing Influence of Machine Learning in Soccer
Additionally, in recent years, the realm of soccer has witnessed a profound transformation. Albeit no longer solely reliant on gut feelings or historical data analysis, soccer prediction AI has stepped onto the field as a formidable contender. With AI football betting tips, AI-powered sports predictions, and predictive modeling for football, it’s clear that artificial intelligence is becoming an integral part of the beautiful game.
1.2. The Need for Accurate Predictions in Soccer
Consequently, why the shift towards AI-driven sports analytics? The answer lies in the insatiable appetite for precision. In addition, in the competitive world of soccer, where outcomes can hinge on the slimmest of margins, having an edge is paramount. AI algorithms for soccer betting promise just that—a competitive edge. Therefore, as we delve deeper into this journey, we’ll explore how these algorithms are reshaping sports forecasting, data-driven predictions, and the very way we view soccer statistics. In conclusion, join us as we embark on an exciting exploration of AI’s role in revolutionizing the world of soccer predictions, and discover how it’s reshaping the way we approach the game we love.
Chapter 2. The Basics of Machine Learning
Furthermore, in the realm of soccer predictions, understanding the fundamentals of machine learning is like mastering the playbook of a championship-winning team. Consequently, in this chapter, we’ll break down the core concepts, from the very definition of machine learning to the diverse array of algorithms driving AI soccer tipsters.
2.1. What is Machine Learning?
Certainly, machine learning, the powerhouse behind AI soccer predictions, is essentially the art of teaching computers to learn from data. Additionally, by analyzing vast amounts of historical soccer data, AI football betting tips become a reality. These algorithms decipher intricate patterns, adapt, and make predictions that transcend human capabilities.
2.2. Types of Machine Learning
Similarly, machine learning isn’t a one-size-fits-all approach; it comes in various flavors, each with its own distinct advantages. Let’s delve into the key types:
2.2.1. Supervised Learning
Basically, in the world of soccer analytics, supervised learning acts as the guiding coach. Albeit it relies on labeled data, where past outcomes are tagged to train the AI. AI-powered sports predictions benefit from this method by drawing on historical match data to predict future results accurately.
2.2.2. Unsupervised Learning
Likewise, unsupervised learning is like letting the AI explore the game on its own. Certainly, it identifies patterns in data without prior guidance. For soccer analytics, this could mean discovering unexpected player dynamics or fan sentiment trends that influence game outcomes.
2.2.3. Reinforcement Learning
Similarly, reinforcement learning, akin to a player honing skills through practice and feedback, involves the AI taking actions in an environment to maximize rewards. Additionally, in the context of sports, this type of learning could be used to optimize betting strategies continuously.
2.3. Machine Learning Soccer Predictions – Algorithms Overview
Moreover, now, let’s explore the lineup of machine learning algorithms driving AI in soccer predictions. Furthermore, these algorithms serve as the tactical formation, shaping the way AI processes data:
- Supervised Algorithms: Algorithms like regression analysis and decision trees are the foundation of AI-driven sports analytics. Additionally, they analyze past performance statistics to make informed future predictions.
- Unsupervised Algorithms: Clustering and association algorithms uncover hidden relationships within soccer data, similarly, helping refine predictive models and soccer statistics.
- Deep Learning: Deep neural networks, inspired by the human brain, are powering breakthroughs in sports data modeling. Likewise, Convolutional Neural Networks (CNNs) and Recurrent Neural Networks (RNNs) are at the forefront, enabling AI to process complex soccer data effectively.
- Ensemble Learning: Just as a strong team comprises different players, ensemble algorithms, such as Random Forest and Gradient Boosting, similarly, combine multiple models to enhance prediction accuracy. In conclusion, with this understanding of machine learning’s fundamentals and the arsenal of algorithms at its disposal, AI in soccer predictions is poised to deliver game-changing insights and AI-powered sports predictions that transcend traditional approaches.
Chapter 3. Machine Learning in Sports
Furthermore, as we continue our exploration into the dynamic world of AI-powered soccer predictions, it’s essential to understand the broader applications of machine learning in the realm of sports. Consequently, in this chapter, we’ll take a closer look at how machine learning algorithms are leaving their mark on the sporting arena, with a specific focus on soccer analytics.
3.1. Applications of Machine Learning in Sports
Indeed, machine learning’s influence in the world of sports is multifaceted, extending well beyond the soccer pitch. Also, it encompasses a wide array of applications, from enhancing athlete performance to optimizing fan engagement. Additionally, let’s touch upon some of the key areas where machine learning shines:
3.2. Machine Learning in Soccer
Albeit within the vast landscape of sports, soccer emerges as a hotbed for machine learning innovation. Furthermore, the beautiful game, with its intricate player dynamics and ever-changing dynamics, presents a fertile ground for AI-driven sports analytics. Here’s a glimpse of how machine learning is making its presence felt in soccer:
3.2.1. Player Performance Analysis
Additionally, player performance analysis is at the heart of soccer analytics. Also, with the help of machine learning algorithms, coaches and analysts gain deeper insights into player behavior, strengths, and weaknesses. These insights, derived from performance statistics, empower teams to make data-driven decisions, whether it’s selecting the ideal starting lineup or fine-tuning training regimes.
3.2.2. Injury Prediction
Likewise, injuries are an unfortunate but inevitable aspect of sports. Consequently, machine learning leverages player data, injury histories, and even external factors like weather conditions to predict injury risks. Also, by identifying patterns and risk factors, AI can assist teams in taking proactive measures to reduce injury occurrences and enhance player longevity.
3.2.3. Match Outcome Prediction
Similarly, the pinnacle of soccer analytics is undoubtedly match outcome prediction. Moreover, with machine learning algorithms fed a steady diet of historical match data, AI becomes a formidable player in forecasting match results. Additionally, fans, bettors, and analysts alike benefit from AI-powered sports predictions that factor in player performance, team dynamics, and countless other variables to provide highly accurate forecasts. As we journey deeper into the world of machine learning in soccer, we’ll witness how these applications come together to reshape the way we view the game. Additionally, from dissecting player performance to predicting match outcomes with uncanny accuracy, the influence of AI in soccer is undeniably transformative.
Chapter 4. Data Collection and Preprocessing for Machine Learning Soccer Predictions
Furthermore, in the realm of AI-powered soccer predictions, data is the lifeblood that fuels the algorithms’ ability to make accurate forecasts. Consequently, in this chapter, we delve into the critical role of data in machine learning, explore the specific methods employed to collect soccer data, and uncover the essential process of data preprocessing—a crucial step in unleashing the potential of AI in sports analytics.
4.1. Importance of Data in Machine Learning Soccer Predictions
Moreover, data is the foundation upon which machine learning algorithms stand. Without a rich and diverse dataset, the power of AI in soccer analytics remains untapped. Additionally, the importance of data lies in its ability to reveal patterns, trends, and insights that would otherwise remain hidden. AI football betting tips, predictive modeling for football, and AI-driven sports analytics all rely on quality data to deliver accurate results.
4.2. Data Collection Methods in Machine Learning Soccer Predictions
Consequently, soccer, with its multifaceted nature, demands a variety of data collection methods to capture every nuance of the game. Here are some prominent methods employed in the world of soccer data collection:
4.2.1. GPS Tracking
Additionally, GPS tracking technology has revolutionized player performance analysis. It provides real-time data on player movement, distance covered, and speed during a match. Also, this wealth of information enables AI to dissect player dynamics and optimize strategies for AI-powered sports predictions.
4.2.2. Video Analysis
Likewise, video analysis serves as the soccer analyst’s playbook. By scrutinizing match footage, analysts extract valuable data points such as player positioning, ball movement, and tactical shifts. Furthermore, AI algorithms ingest this visual data to enhance their understanding of the game, contributing to more accurate predictive modeling for football.
4.3. Data Preprocessing for ML
Additionally, while data is a treasure trove of insights, it often arrives in a raw and unstructured form. Data preprocessing is the key to refining this raw material into a format that machine learning algorithms can digest effectively.
4.3.1. Cleaning and Formatting
Consequently, cleaning involves identifying and rectifying errors or inconsistencies in the data. Formatting ensures that data is in a standardized, machine-readable format. Also, this step is pivotal in ensuring that AI football betting tips and other predictive models receive clean, reliable input.
4.3.2. Feature Selection
Furthermore, feature selection is akin to selecting the most valuable players for a starting lineup. It involves choosing the most relevant data attributes (features) for analysis while discarding less informative ones. Moreover, this process streamlines the dataset, enabling AI algorithms to focus on what truly matters in soccer analytics. In our journey to comprehend the inner workings of AI-powered soccer predictions, data collection and preprocessing emerge as foundational pillars. Without the right data collected through sophisticated methods and meticulously preprocessed to perfection, AI’s potential in transforming the world of soccer analytics remains untapped.
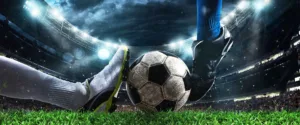
Chapter 5. Machine Learning Models in Soccer Predictions
Additionally, in the realm of AI-driven soccer predictions, the choice of machine learning models is akin to selecting the right formation for a championship-winning team. In this chapter, we’ll delve into the key players—decision trees, random forests, support vector machines, neural networks, and gradient boosting—and explore how each contributes to the world of AI-powered sports predictions.
5.1. Decision Trees
Furthermore, decision trees, much like a coach’s playbook, provide a structured approach to decision-making. These models break down complex decisions into a series of simple questions, allowing AI to navigate the soccer analytics landscape efficiently. Decision trees are valuable players in the world of AI football betting tips, helping to dissect historical data and extract patterns for future predictions.
5.2. Random Forest
Likewise, random forests, a team of decision trees working in harmony, bring robustness to AI-powered sports predictions. By combining multiple decision trees, random forests reduce the risk of overfitting and enhance prediction accuracy. They’re the defensive line, safeguarding against erroneous outcomes in the world of soccer prediction AI.
5.3. Support Vector Machines
Consequently, support vector machines (SVMs) act as the midfield maestros in AI-driven sports analytics. SVMs excel in separating data points into distinct classes. In the context of soccer analytics, they’re adept at distinguishing between winning and losing outcomes, making them invaluable in match outcome prediction.
5.4. Neural Networks
Additionally, neural networks, inspired by the human brain, are the star forwards in the AI soccer tipsters’ lineup. These deep learning models process complex, multidimensional data to uncover intricate patterns. Convolutional neural networks (CNNs) shine in image analysis, while recurrent neural networks (RNNs) capture temporal dependencies, enhancing player performance analysis and sports data modeling.
5.5. Gradient Boosting
Furthermore, gradient boosting, the tactical strategist of machine learning models, elevates predictive modeling for football to new heights. It iteratively refines predictions, learning from past mistakes to make increasingly accurate forecasts. With each iteration, gradient boosting becomes more adept at capturing the nuances of soccer, contributing to the precision of AI-powered sports predictions. As we explore the roles of these machine learning models in soccer analytics, we’ll witness how each model adds its unique strengths and strategies to the game. Together, they form a formidable squad that not only enhances the accuracy of soccer predictions but also redefines how we approach the beautiful game.
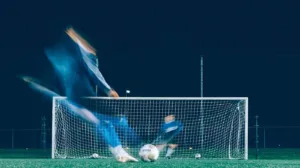
Chapter 6. Case Studies in Machine Learning Soccer Predictions
Additionally, in our journey through the fascinating world of AI-powered soccer predictions, it’s time to shift our focus to real-world applications. In this chapter, we’ll delve into two compelling case studies—a deep dive into injury prediction and a closer look at match outcome prediction. These case studies will illuminate the practicality and effectiveness of AI algorithms in soccer analytics.
6.1. Injury Prediction Case Study
Injuries can significantly impact a team’s performance and player careers. AI’s role in injury prediction is transformative, as showcased in this case study.
6.1.1. Data Collection and Features
Furthermore, we’ll explore the intricate process of data collection for injury prediction, including the critical features extracted from player data such as GPS tracking, video analysis, and historical injury records. These features serve as the playbook for AI to predict potential injuries.
6.1.2. Model Selection and Training
Moreover, the selection of appropriate machine learning models and the training process are key plays in this case study. We’ll reveal which models shine in injury prediction and how they are fine-tuned using historical data to achieve optimal performance.
6.1.3. Results and Accuracy
Consequently, in the world of sports forecasting, accuracy is the MVP. We’ll delve into the results generated by AI algorithms in predicting injuries, showcasing their ability to provide actionable insights for coaches and medical staff.
6.2. Match Outcome Prediction Case Study
Predicting match outcomes is the pinnacle of soccer analytics. In this case study, we’ll explore how AI algorithms excel in this critical aspect of sports predictions.
6.2.1. Data Sources and Variables
Additionally, we’ll examine the diverse data sources and variables that fuel AI models for match outcome prediction. These include player performance data, team statistics, historical match records, and even external factors like weather conditions and venue history.
6.2.2. Model Building and Evaluation
Furthermore, the process of building AI models for match outcome prediction involves selecting the right features, model selection, and rigorous evaluation. We’ll uncover the strategies that lead to high accuracy in forecasting game results.
6.2.3. Insights from Predictions
Likewise, we’ll explore the insights generated from match outcome predictions. These insights can be invaluable for fans, bettors, and team strategists, offering a deeper understanding of the dynamics at play and helping them make more informed decisions. Through these case studies, we’ll witness the real-world impact of AI-driven sports analytics in soccer. From preventing injuries and optimizing player performance to accurately forecasting match outcomes, AI algorithms are rewriting the rules of the beautiful game, ushering in a new era of precision and understanding.
Chapter 7. Challenges and Ethical Considerations for Machine Learning Soccer Predictions
Furthermore, as we venture deeper into the realm of AI-powered soccer predictions, it’s essential to acknowledge the hurdles and ethical dimensions that come into play. In this chapter, we confront the ethical concerns surrounding predictive analytics and grapple with the challenges that arise when implementing machine learning in the world of soccer.
7.1. Ethical Concerns in Predictive Analytics
Additionally, predictive analytics, while a powerful tool, raises important ethical questions. We’ll delve into these concerns, including issues of privacy, data security, and fairness. Ethical considerations are vital in ensuring that AI in soccer predictions respects the rights and well-being of players, coaches, and fans alike.
7.2. Challenges in Implementing ML in Soccer
Moreover, the implementation of machine learning in soccer comes with its own set of challenges. We’ll explore the obstacles faced by teams, analysts, and organizations as they adopt AI algorithms for soccer analytics. These challenges encompass data quality, model interpretability, and the need for skilled personnel to navigate the ever-evolving landscape of AI-driven sports analytics. Consequently, navigating the ethical considerations and addressing the challenges is pivotal in harnessing the full potential of AI in soccer predictions while upholding the values and integrity of the beautiful game. In this chapter, we’ll grapple with these complex issues, paving the way for a responsible and ethically sound integration of machine learning into the world of soccer.
Chapter 8. Future of Machine Learning in Soccer
Furthermore, in our concluding chapter, we gaze into the crystal ball to envision the exciting future of machine learning in soccer. The potential for innovation and advancement in the field of AI-powered soccer predictions is boundless, promising to reshape not only the game itself but also its impact on the world of sports.
8.1. Innovations and Advancements in Machine Learning Soccer Predictions
Additionally, the future holds a treasure trove of innovations in machine learning for soccer. We’ll explore emerging technologies, such as real-time analytics, augmented reality for player performance analysis, and the integration of AI-driven sports predictions into fan engagement platforms. These innovations will redefine how we experience and interact with the game.
8.2. Potential Impact on Soccer as a Sport
Moreover, machine learning’s influence on soccer extends far beyond the pitch. We’ll reflect on the potential impact of AI-powered soccer predictions on the sport as a whole. This includes improved player performance, enhanced coaching strategies, fan engagement through personalized experiences, and the evolution of sports betting. Consequently, as we conclude our exploration of the future of machine learning in soccer, we’ll recognize the transformative potential of AI algorithms. They are not merely tools for predicting match outcomes; they are catalysts for innovation, pushing the boundaries of what’s possible in the world of sports. The future of soccer is bright, powered by the limitless possibilities of AI-driven sports analytics.
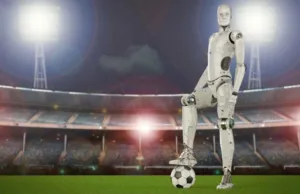
Chapter 9. Frequently Asked Questions (FAQs) About Machine Learning Soccer Predictions
Moreover, in this section, we address some common questions that often arise when delving into the world of machine learning in soccer predictions.
9.1. What is the role of machine learning in injury prevention?
Certainly, machine learning plays a crucial role in injury prevention by analyzing player data, historical injury records, and various external factors. AI algorithms can identify patterns and risk factors, helping teams take proactive measures to reduce the likelihood of injuries and keep players in peak condition.
9.2. How do machine learning models predict match outcomes?
Additionally, machine learning models predict match outcomes by analyzing a multitude of factors, including player performance data, team statistics, historical match records, and external variables like weather conditions. These models use these features to make informed predictions about which team is likely to win, lose, or draw.
9.3. Are there any privacy concerns with player tracking data?
Consequently, yes, privacy concerns can arise with player tracking data. The collection of personal and performance data raises questions about data ownership, consent, and the potential for misuse. Ethical considerations and data protection measures are essential to address these concerns and protect the privacy of players.
9.4. Can machine learning be used to scout young talent in soccer?
Indeed, machine learning can be a valuable tool for scouting young talent in soccer. By analyzing player performance metrics and comparing them to historical data, AI algorithms can help identify promising young players who may have the potential to excel in the sport.
9.5. What are the limitations of machine learning soccer predictions?
Nevertheless, machine learning in soccer predictions, while powerful, has its limitations. These limitations include the need for high-quality and comprehensive data, the potential for bias in data and models, and the challenges of accurately predicting unexpected events or game-changing moments. Additionally, AI models are only as good as the data they are trained on, and they may struggle with rapidly evolving player dynamics or rule changes in the sport. It’s essential to recognize these limitations while leveraging the strengths of AI in soccer analytics.
Chapter 10. Conclusion
Additionally, as we draw the final whistle on our journey through the world of machine learning in soccer, it’s time to reflect on the remarkable strides that AI has taken in reshaping the way we understand and engage with the beautiful game.
10.1. Recap of Machine Learning Soccer Predictions
Throughout this exploration, we’ve witnessed the transformative power of machine learning in soccer predictions. Furthermore, from dissecting player performance and injury prevention to forecasting match outcomes with precision, AI algorithms have become invaluable players in the world of sports analytics. The role of data, model selection, and ethical considerations have all come into focus as we’ve uncovered the intricate workings of AI-driven soccer analytics.
10.2. Looking Ahead: The Exciting Future of Machine Learning Soccer Predictions
Consequently, but this is just the beginning. The future of soccer predictions is ablaze with innovation and potential. Emerging technologies, real-time analytics, and personalized fan experiences are set to redefine the way we engage with the sport. As AI continues to evolve, it promises to elevate soccer not just as a game but as an immersive and data-driven experience for fans, players, and teams alike. So, what’s next? Dive deeper into the world of soccer predictions and stay ahead of the game by exploring FootCast’s other blog posts. Additionally, sign up for our newsletter to receive the latest insights, trends, and breakthroughs in AI-driven sports analytics. Whether you’re a passionate fan or a data-savvy analyst, together, we’ll continue to unravel the exciting future of soccer powered by machine learning. Thank you for joining us on this thrilling journey.
Your point of view caught my eye and was very interesting. Thanks. I have a question for you.
Thank you so much. Please reach us via hello@footcast.net