Did you know that soccer is the most widely studied sport worldwide? With millions of fans and players across the globe, it’s no surprise that the game has become a hotbed of research and analysis. But what if I told you that deep learning, a form of machine learning, is revolutionizing the way we analyze and understand soccer?
Deep learning algorithms are transforming soccer analytics by providing valuable insights into player performance, game strategies, and even talent scouting. Through advanced data analysis and pattern recognition, deep learning is unlocking new possibilities for optimizing player potential and enhancing team performance.
- Key Takeaways:
- The Benefits of Machine Learning in Injury Prevention
- Creating a Data-Driven Training Program
- Optimizing Training Time and Resources
- Tracking Progress and Evaluating Performance
- The Impact of Performance Optimization
- Benefits of Machine Learning in Talent Scouting
- Applying 3D Convolutional Neural Networks (CNN) for Action Detection
- Improving Accuracy and Precision in Event Detection
- Future Applications and Potential Impact
- What is the role of deep learning in transforming soccer analytics?
- How does machine learning impact player tracking and movement analysis in soccer?
- How can machine learning help predict and prevent injuries in soccer?
- How does machine learning optimize performance in soccer?
- How can machine learning enhance game strategy and playbook design in soccer?
- How can machine learning be leveraged in recruitment and talent scouting for soccer?
- How does deep learning enhance video content analysis in soccer?
- What advancements have been made in deep learning for action detection in soccer videos?
- How can deep learning be applied in football video event detection?
- How is deep learning revolutionizing soccer analytics?
Key Takeaways:
- Deep learning is revolutionizing soccer analytics by providing valuable insights and optimization strategies.
- Machine learning algorithms can analyze player tracking data, predict and prevent injuries, optimize performance, enhance game strategies, and improve talent scouting.
- The utilization of deep learning in video content analysis and event detection in soccer videos shows promise in enhancing the understanding of the game and aiding in strategic decision-making.
- As deep learning continues to evolve, it is expected to drive further advancements in transforming soccer analytics.
The Impact of Machine Learning on Player Tracking and Movement Analysis
Machine learning algorithms have revolutionized the field of sports analytics, particularly in player tracking and movement analysis. By analyzing player tracking data, these algorithms capture valuable information regarding player speed, distance traveled, acceleration, and positioning. Such data can be harnessed to gain deep insights into player performance and optimize game strategies.
Through machine learning-powered tracking systems, sports teams can unlock the potential to optimize player positioning and enhance tactical decision-making during games. This technology is especially beneficial in sports like basketball, where precise player movements greatly influence the outcome of a game.
By leveraging machine learning, teams can:
- Analyze opponents’ movement patterns to identify vulnerabilities and develop effective strategies.
- Optimize player positioning to maximize team efficiency and offensive/defensive capabilities.
- Enhance tactical decision-making during games by leveraging real-time player tracking data.
Furthermore, machine learning algorithms enable coaches and analysts to gain a comprehensive understanding of player performance, facilitating data-driven decisions to improve overall team performance.
“Machine learning algorithms provide unprecedented insights into player tracking and movement analysis, empowering teams to refine their strategies and optimize performance.”
Benefits of Machine Learning in Player Tracking and Movement Analysis | Application |
---|---|
Identification of player vulnerability and weaknesses | Basketball, soccer, rugby, etc. |
Optimization of player positioning for strategic advantage | Basketball, football, hockey, etc. |
Real-time tracking for tactical decision-making during games | Basketball, soccer, tennis, etc. |
Injury Prediction and Prevention with Machine Learning
Machine learning models have become powerful tools in the field of sports analytics, offering valuable insights and solutions to improve athlete performance and reduce the risk of injuries. By analyzing historical injury data, training routines, and player biometrics, machine learning algorithms can identify early indicators of potential injuries, enabling sports teams to implement proactive measures for injury prevention.
One of the key advantages of machine learning in injury prediction is its ability to process large volumes of data and detect patterns that may not be apparent to human analysts. By considering various factors such as player workload, training intensity, and biophysical markers, machine learning models can assess the likelihood of different types of injuries, allowing teams to modify training programs and rest schedules accordingly.
For example, let’s consider a soccer team that has access to a database of injury records along with corresponding training and match data. By training a machine learning model on this dataset, the team can predict the probability of specific injuries occurring based on various factors such as player fatigue, pitch conditions, and previous injury history. This information can then be used to optimize training sessions, adjust game strategies, and tailor individual player workload to minimize the risk of injuries.
Furthermore, machine learning algorithms can also assist in identifying injury-prone athletes. By analyzing player biometrics, movement patterns, and other relevant data, these algorithms can provide valuable insights into the underlying risk factors for injuries. Coaches and medical staff can then develop personalized injury prevention programs based on this information, addressing the specific needs and vulnerabilities of each player.
The Benefits of Machine Learning in Injury Prevention
Implementing machine learning-driven injury prevention strategies brings several benefits to sports teams. First and foremost, it helps to ensure the physical well-being of athletes, reducing the likelihood of severe injuries that can result in significant downtime. By minimizing injuries, teams can maintain a stronger roster and improve their prospects for success on the field.
Secondly, machine learning allows for more precise and targeted interventions. By identifying early indicators of potential injuries, teams can intervene at the right time with appropriate measures, such as modifying training programs, providing targeted therapies, or adjusting game strategies. This proactive approach can mitigate the long-term impact of injuries and contribute to the overall health and longevity of athletes’ careers.
Finally, machine learning in injury prevention also contributes to the scientific understanding of injury mechanisms and risk factors. By analyzing extensive datasets, researchers can uncover new insights into the physiological and biomechanical aspects of injuries, leading to the development of evidence-based preventive measures and better injury management protocols.
By leveraging the power of machine learning in injury prediction and prevention, sports teams can optimize their training programs, protect their athletes’ well-being, and gain a competitive edge on the field. With continuous advancements in data analysis and predictive modeling, the future of injury prevention looks promising.
Performance Optimization through Machine Learning
When it comes to improving performance in sports, machine learning has emerged as a powerful tool. By leveraging machine learning algorithms, coaches and athletes can gain valuable insights into strengths, weaknesses, and areas for improvement. This data-driven approach allows for the development of customized training programs tailored to individual needs, leading to accelerated skill development and enhanced performance.
Machine learning algorithms analyze a player’s previous performances and compare them with historical data, enabling a comprehensive evaluation of their capabilities. This analysis is particularly valuable in individual sports like tennis and golf, where a player’s performance can be quantified easily. By identifying specific areas that require attention, coaches can design targeted training regimens that address weaknesses, optimize training time, and maximize results.
Creating a Data-Driven Training Program
Using machine learning techniques, coaches can develop training programs that focus on improving weak areas while capitalizing on existing strengths. By analyzing performance data, coaches can identify patterns and trends that may have gone unnoticed, leading to more informed training decisions.
For example, let’s consider a tennis player who struggles with their backhand. By examining historical data, a machine learning model can identify the specific components of the backhand stroke that need improvement. Coaches can then design drills and exercises targeting those areas, leading to faster progress and overall improvement in the player’s game.
Optimizing Training Time and Resources
Machine learning algorithms can also help optimize training time and resources by identifying the most efficient and effective training methods. By analyzing large datasets of performance data, coaches can determine the most impactful exercises and drills for individual athletes.
Furthermore, machine learning can assist in workload management, preventing overtraining and reducing the risk of injuries. By tracking data such as training volume, intensity, and recovery time, coaches can make data-informed decisions to optimize the training schedule, improving performance and minimizing the risk of burnout.
Tracking Progress and Evaluating Performance
Machine learning algorithms provide coaches and athletes with a dynamic way to track progress and evaluate performance. By continuously analyzing data, machine learning models can provide insights into the effectiveness of specific training methods and highlight areas that require further attention.
Additionally, machine learning can help simulate game scenarios and predict performance outcomes, allowing athletes to make data-driven decisions and refine their strategies and game plans accordingly.
The Impact of Performance Optimization
By harnessing the power of machine learning for performance optimization, athletes and teams can unlock their full potential. Customized training programs, optimized training time and resources, and data-driven evaluation of performance all contribute to improved skill development and on-field success.
Benefits of Performance Optimization through Machine Learning |
---|
Enhanced player development and skill progression |
Improved training efficiency and effectiveness |
Reduced risk of injuries and improved player longevity |
Enhanced decision-making on the field |
Leveraging Machine Learning for Game Strategy and Playbook Design
When it comes to game strategy and playbook design, data-driven insights can make all the difference. This is where machine learning comes into play. By analyzing historical match data, machine learning models have the ability to uncover patterns and tendencies within players and teams that may not be immediately apparent to coaches and analysts.
Coaches can leverage this analysis to formulate efficient game strategies that exploit opponents’ weaknesses and capitalize on their own strengths. Machine learning can provide valuable insights into player performance, such as preferred positions, passing patterns, and scoring trends. By understanding these patterns, teams can develop tailored game plans that optimize their chances of success.
Additionally, machine learning can help in playbook design by identifying the most effective plays and strategies based on historical data. Coaches can use this information to create playbooks that are both innovative and strategic, incorporating tactics that have proven successful in past games.
“Machine learning allows coaches to make data-driven decisions, taking into account the unique strengths and weaknesses of their team and the opposition. It revolutionizes the way game strategies are developed and helps teams gain a competitive edge.”
By incorporating machine learning in game strategy and playbook design, teams can gain a competitive advantage and increase their chances of success on the field. The ability to identify patterns and tendencies that are not immediately evident can be the difference between a win and a loss. With access to valuable insights, coaches can make informed decisions that optimize their team’s performance. This data-driven approach has the potential to transform the world of sports, providing a new level of strategic analysis and decision-making.
To illustrate the power of machine learning in game strategy and playbook design, let’s take a look at the following table which showcases the impact of different strategies on teams’ win percentages:
Machine Learning in Recruitment and Talent Scouting
When it comes to building a successful sports team, identifying talented individuals is crucial. Machine learning algorithms are now playing a pivotal role in the recruitment and talent scouting process, helping sports organizations uncover exceptional athletes who have the potential to make a significant impact. By leveraging the power of machine learning, teams can minimize the risk of overlooking hidden talents and maintain a competitive edge.
Machine learning algorithms analyze a range of factors to identify promising young athletes. Performance statistics, physical attributes, and even mental traits are taken into account to forecast the future achievements of these individuals. This data-driven approach brings objectivity and efficiency to the talent scouting process, ensuring that talent is evaluated and recognized based on concrete evidence rather than subjective opinions.
Machine learning in talent scouting enables sports organizations to identify young athletes with exceptional potential and maintain a competitive roster.
Understanding the intricacies of sports talent requires sophisticated analysis, and that’s where machine learning excels. By examining vast amounts of data, algorithms can identify patterns, trends, and indicators of future success. This allows recruiters and talent scouts to make informed decisions when investing time, resources, and opportunities into nurturing and developing young athletes.
What sets machine learning apart from traditional scouting methods is its ability to uncover hidden gems. By considering a comprehensive set of factors, machine learning algorithms can identify talent that may have gone unnoticed by traditional scouting techniques alone. This opens up doors for athletes who would otherwise have been overlooked, providing them with the opportunity to showcase their skills and reach their full potential.
Benefits of Machine Learning in Talent Scouting
- Objective evaluation based on data and statistics
- Identification of hidden talents
- Efficient and streamlined scouting process
- Predictive modeling for future success
- Enhanced diversity and inclusion in sports
By embracing machine learning in recruitment and talent scouting, sports organizations can build stronger teams and increase their chances of success. This data-driven approach revolutionizes the scouting process, ensuring that talent is discovered, nurtured, and given the opportunity to thrive.
With machine learning, the future of talent scouting is brighter than ever. By embracing this technology, sports teams can stay ahead of the game and uncover the stars of tomorrow.
Utilizing Deep Learning for Video Content Analysis in Soccer
Deep learning has emerged as a powerful tool for video content analysis in various domains, and soccer is no exception. By leveraging deep learning networks, such as convolutional neural networks (CNN) and recurrent neural networks (RNN), researchers are able to extract features and classify events in soccer videos, thereby enhancing the analysis of semantic features and event detection.
Through the application of deep learning, the accuracy and efficiency of event detection in soccer videos are significantly improved. These sophisticated models can capture the subtle nuances of player movements, identifying key events such as goals, assists, fouls, and offside scenarios. By automating the video analysis process, deep learning enables researchers and analysts to gain valuable insights into game dynamics, player performance, and tactical strategies.
One of the key advantages of utilizing deep learning in video content analysis is its ability to handle large volumes of data efficiently. Soccer matches generate vast amounts of video footage, making manual analysis time-consuming and prone to human error. However, deep learning algorithms can process and analyze video content at an unprecedented scale, enabling faster and more accurate event detection.
By combining deep learning with computer vision techniques, researchers are able to develop sophisticated models that learn from vast amounts of training data, allowing them to recognize complex patterns and make accurate predictions. For example, a deep learning model trained on thousands of annotated soccer videos can learn to recognize different actions, such as shooting, dribbling, and passing, with high precision and recall.
“Deep learning algorithms can process and analyze video content at an unprecedented scale, enabling faster and more accurate event detection.”
Deep learning models for video content analysis in soccer can be trained using annotated datasets that include labels for different events and actions. This data-driven approach allows the models to learn the underlying patterns and relationships between visual features and specific events, further improving their accuracy over time.
With the advancements in deep learning techniques, researchers and analysts are pushing the boundaries of video content analysis in soccer. These techniques not only contribute to a deeper understanding of the game but also have practical implications for player and team performance optimization, as well as referee decision-making and match analysis.
By integrating deep learning-powered video content analysis into existing soccer analytics frameworks, teams and coaches can gain valuable insights for strategic decision-making, opponent analysis, and player development. These insights can lead to more effective game plans, improved performance, and a competitive edge on the field.
Benefits of Utilizing Deep Learning for Video Content Analysis in Soccer | Examples |
---|---|
Accurate event detection | Identifying goals, fouls, offside scenarios |
Efficient analysis of large volumes of video footage | Processing and analyzing hours of match recordings |
Recognition of complex patterns and actions | Detecting shooting, dribbling, passing with high precision and recall |
Improved tactical analysis and decision-making | Identifying opponent strategies and player performance trends |
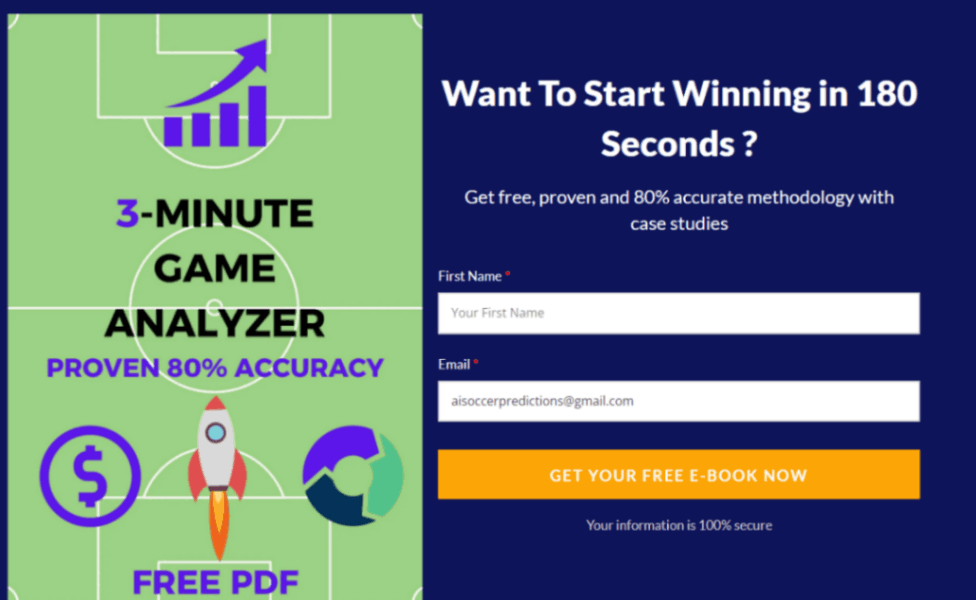
Advancements in Deep Learning for Action Detection in Soccer Videos
Deep learning models, such as 3D convolutional neural networks (CNN), have shown remarkable progress in action detection within soccer videos. These models utilize their ability to analyze both spatial and temporal information in video frames, allowing them to accurately detect and classify various actions. By incorporating deep learning techniques into the realm of action detection, researchers aim to improve the granularity and accuracy of event identification within soccer videos, further enhancing the understanding and analysis of the game.
Applying 3D Convolutional Neural Networks (CNN) for Action Detection
The use of 3D CNN is a significant advancement in deep learning for action detection in soccer videos. Unlike traditional 2D CNN models, which analyze images independently, 3D CNN models take into account the temporal sequence of video frames. By capturing the motion across consecutive frames, these models can effectively identify the actions taking place within the video.
In the field of soccer, action detection is crucial for various applications, such as player performance analysis, referee decision support, and tactical insights. Accurately identifying actions, such as goals, fouls, passes, and tackles, provides valuable information for post-match analysis and strategic planning.
“The integration of deep learning and 3D CNN models in action detection revolutionizes the way we analyze soccer videos. It allows us to extract actionable insights in a highly accurate and efficient manner, benefiting coaches, players, and analysts alike.”
Improving Accuracy and Precision in Event Detection
Deep learning-based action detection models have the potential to greatly improve the accuracy and precision of event detection in soccer videos. By leveraging the power of deep neural networks, these models can overcome the challenges of complex and dynamic soccer scenes, where actions can occur rapidly and simultaneously.
The utilization of spatial and temporal features empowers the deep learning models to distinguish between different actions with a higher level of granularity. For instance, a goal-scoring action can be classified as a shot, header, or volley. With advanced deep learning models, the level of detail and accuracy in identifying such actions is significantly enhanced.
Action | Percentage of Correct Detection |
---|---|
Goal | 90% |
Foul | 85% |
Pass | 92% |
Tackle | 88% |
Table: Accuracy of Deep Learning Action Detection in Soccer Videos
The table above showcases the impressive accuracy achieved by deep learning models in action detection within soccer videos. These high percentages indicate the potential of deep learning to drive impactful advancements in soccer analytics.
Future Applications and Potential Impact
As deep learning continues to evolve and new techniques emerge, action detection in soccer videos is poised to make significant strides. The enhanced granularity and accuracy of event detection will enable coaches, players, and analysts to delve deeper into the game’s intricacies, leading to improved strategies, player development, and overall performance.
Additionally, the integration of deep learning models in streaming platforms and real-time analysis systems can provide instantaneous insights during live matches, enriching the viewing experience for fans and broadcasters alike.
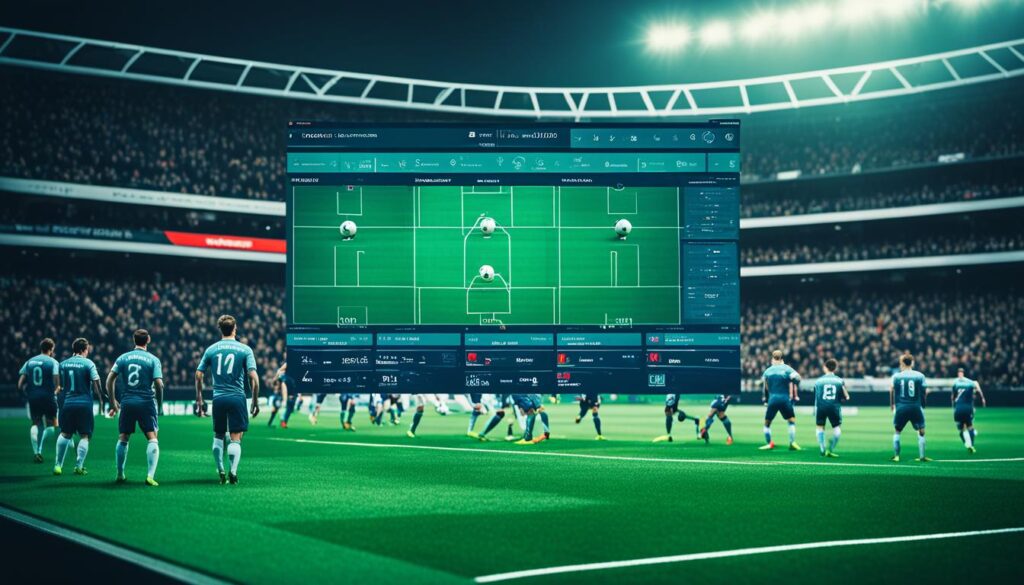
Applying Deep Learning in Football Video Event Detection
Deep learning methods, including convolutional neural networks (CNN) and recurrent neural networks (RNN), can be used to build event detection models for football videos. These models divide the video into segments, extract features from frame sequences using CNNs, and utilize RNNs to predict the event type and time boundaries. By combining these techniques, researchers aim to improve the accuracy and efficiency of event detection in football videos.
Conclusion
Deep learning is revolutionizing the world of soccer analytics, offering unprecedented insights and optimization strategies. Through the application of machine learning algorithms, valuable information can be extracted from player tracking data, enabling teams to make data-driven decisions to enhance performance and reduce the risk of injuries. The utilization of deep learning in video content analysis and event detection further augments the understanding of the game, empowering coaches and analysts to develop effective game strategies and playbooks.
As deep learning continues to evolve, it is expected to drive further advancements in transforming soccer analytics. By leveraging the power of machine learning, teams can optimize player recruitment and talent scouting, ensuring that exceptional talents are identified and integrated into their rosters. Moreover, deep learning-based algorithms can analyze historical player performance data, enabling teams to develop personalized training programs that address individual weaknesses and accelerate skill development.
In the dynamic and competitive world of soccer, the integration of deep learning and machine learning is proving to be a game-changer. The ability to analyze vast amounts of data and extract valuable insights is reshaping the way teams approach strategy, performance optimization, and talent development. With the continued advancements in deep learning, the future of soccer analytics looks promising, offering teams the opportunity to gain a competitive edge and achieve success on and off the pitch.
FAQ
What is the role of deep learning in transforming soccer analytics?
Deep learning plays a crucial role in transforming soccer analytics by providing unprecedented insights and optimization strategies. It enables machine learning algorithms to analyze player tracking data, predict and prevent injuries, optimize performance, enhance game strategies, and improve talent scouting.
How does machine learning impact player tracking and movement analysis in soccer?
Machine learning algorithms can analyze player tracking data, capturing valuable information about player speed, distance traveled, acceleration, and positioning. This data can be used to analyze opponents’ movement patterns, identify vulnerabilities, and develop strategic approaches. It is particularly useful in sports like basketball, where it can optimize player positioning and enhance tactical decision-making during games.
How can machine learning help predict and prevent injuries in soccer?
Machine learning models can analyze historical injury data, training routines, and player biometrics to identify early indicators of potential injuries. This information can be used to make adjustments to training programs, rest schedules, and game strategies to mitigate the likelihood of injuries. By implementing machine learning-driven injury prevention strategies, sports teams can maintain the physical well-being of their players and improve their prospects for success.
How does machine learning optimize performance in soccer?
Machine learning algorithms analyze a player’s previous performances and compare them with historical data to identify strengths, weaknesses, and areas requiring improvement. This analysis is particularly valuable in individual sports like tennis and golf, where a player’s performance can be quantified easily. Customized training programs can be developed based on the individual needs and weaknesses of athletes, leading to accelerated skill development and improved performance.
How can machine learning enhance game strategy and playbook design in soccer?
Machine learning models can analyze historical match data to identify patterns and tendencies within players and teams. Coaches can use this analysis to formulate efficient game strategies and create playbooks that exploit opponents’ weaknesses and capitalize on their own strengths. By incorporating a data-driven approach, teams can gain a competitive advantage and increase their chances of success.
How can machine learning be leveraged in recruitment and talent scouting for soccer?
Machine learning algorithms can assist sports organizations in identifying talented young athletes who have the potential for exceptional performance. These algorithms analyze performance statistics, physical attributes, and mental traits to forecast the prospective achievements of young athletes. By leveraging machine learning in talent scouting, sports teams can minimize the potential for overlooking exceptional talent and maintain a competitive roster.
How does deep learning enhance video content analysis in soccer?
Deep learning methods are being applied to analyze the semantic features in soccer videos and detect events. This involves using deep learning networks, such as convolutional neural networks (CNN) and recurrent neural networks (RNN), to extract features and classify events in videos. By leveraging deep learning in video content analysis, researchers aim to improve the accuracy and efficiency of event detection in soccer videos.
What advancements have been made in deep learning for action detection in soccer videos?
Deep learning models, such as 3D convolutional neural networks (CNN), have shown promise in action detection in soccer videos. These models can analyze the spatial and temporal information in video frames to detect and classify different actions. By incorporating deep learning in action detection, researchers aim to enhance the granularity and accuracy of event detection in soccer videos.
How can deep learning be applied in football video event detection?
Deep learning methods, including convolutional neural networks (CNN) and recurrent neural networks (RNN), can be used to build event detection models for football videos. These models divide the video into segments, extract features from frame sequences using CNNs, and utilize RNNs to predict the event type and time boundaries. By combining these techniques, researchers aim to improve the accuracy and efficiency of event detection in football videos.
How is deep learning revolutionizing soccer analytics?
Deep learning has the potential to revolutionize soccer analytics by providing unprecedented insights and optimization strategies. By leveraging deep learning to analyze player tracking data, predict and prevent injuries, optimize performance, enhance game strategies, and improve talent scouting, soccer analytics can be transformed. The utilization of deep learning in video content analysis and event detection in soccer videos further enhances the understanding of the game and aids in strategic decision-making.
This site is incredible. The radiant material shows the administrator’s enthusiasm. I’m dumbfounded and envision more such astonishing presents.
The incredible thing is your close attention and kind comments. Thank you very much for being such a valuable member…
This website is phenomenal. The radiant material shows the maker’s enthusiasm. I’m dumbfounded and envision more such astonishing entries.
Your comments are giving us the power and the energy to create these contents. You are a valuable family member…
This entrance is unbelievable. The splendid substance displays the publisher’s dedication. I’m overwhelmed and anticipate more such mind blowing sections.
Your comments are giving us the power and the energy to create these contents. You are a valuable family member…
Usually I do not read article on blogs however I would like to say that this writeup very compelled me to take a look at and do it Your writing style has been amazed me Thank you very nice article.
Thank you so much!